Help Stop SOAR Abuse
Risk and complexity are on the rise from use cases that automation was never intended to support
Don’t get me wrong—Security Orchestration, Automation, and Response (SOAR) is an increasingly valuable part of security operations. Also, this isn’t a “SOAR is dead” post, although hyperautomation is certainly a cooler name. This is about using the right tool for the job. Read on to learn how SOC teams unintentionally take on long-term risk and complexity by using (or abusing) SOAR for detection engineering use cases.
SOAR Picks Up The SIEM Slack
Security automation is a best practice for reducing the burden of manual SOC processes. For example, many SOCs have an automated playbook that sorts through employee-reported phishing emails, checks elements against threat intelligence, and yanks any related emails from company mailboxes. Should the same automation approach be applied to threat detection?
The problem starts when detection requirements involve uncollected activity logs, a common situation given SOCs tend to have less than half their data in the SIEM. Visibility gaps become detection gaps. The workaround goes like this: the SOAR reaches out to a data source API, asks a question, and notifies the team based on the result. It sounds workable, and I’ve spoken with several security leaders considering SOAR to solve their SIEM detection gaps.
Another way SOAR compensates for SIEM shortcomings is by attempting to reduce alert noise. Many SIEM deployments generate far more alerts than the SOC can process, resulting in missed threats and a generally bummer experience. Some teams have taken to automation playbooks as a correlation layer for noisy atomic alerts. The hope is for SOAR to close out a significant portion of alerts or tickets based on automated steps before they reach the analyst’s queue.
Rubber Bands, Paperclips, and Playbooks
We just reviewed some compelling-sounding applications for security automation. The effectiveness of these use cases in an enterprise production environment is another story. Since SOAR solutions were designed to help with manual tasks downstream from the SIEM, they have critical gaps affecting teams trying to MacGyver a threat detection solution.
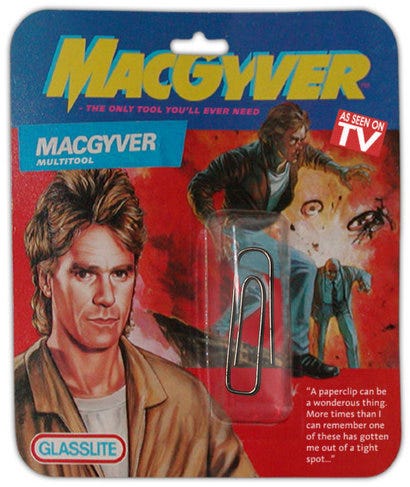
One challenge with using SOAR as a rule engine stems from how logs are turned into alerts. Logs that match detection logic are often clumped together, with many matching events happening around the same time. For example, a password-spraying attack involving thousands of attempts in a few minutes. That’s why SIEM rule engines include sophisticated deduplication and debouncing mechanisms to merge related detections and prevent issuing a succession of identical alerts.
Also, detection engineering has matured recently, including through alignment with the MITRE ATT&CK framework. Security organizations speak in ATT&CK terms and measure coverage against ATT&CK-mapped tactics and techniques (TTPs). A team that makes up for SIEM shortcomings by breaking out some of its detections to the SOAR cannot effectively track and prioritize its detection coverage. The result is a detection engineering mess.
SOC metrics suffer because the SOAR does not report key SIEM stats such as top offenders, mean time to detect, and false positive rates. As with MITRE ATT&CK coverage, these metrics are not supported by solutions not designed for threat detection. This impacts the SOC's overall maturity, especially that of the detection engineering program, at a time when improving maturity is a priority for many CISOs.
Finally, security analysts are directly impacted by SOAR abuse when they find themselves on the hook to build extensive detection content from scratch. Unlike SIEM products with frequently updated content packs, SOAR providers do not consider threat detection coverage in the scope of their solution. The customer team must then bear the burden of researching emerging attacks, developing detection logic, and testing it for false positives and negatives. All this is especially time-consuming when performed outside of a purpose-built detection engineering workbench.
The Right Tool for the Job
Using the intended tool for each phase in the SOC lifecycle can avoid workarounds that cause a detection engineering mess. This is especially clear as the traditional SIEM continues to unbundle (or Splunkbundle). From data collection to analytics to automation, reliance on best-of-breed tooling removes the motivation to hack unintended use cases.
For data collection, owning the data pipeline and the data lake removes the aforementioned limitations on visibility. With 80% lower costs and always-hot retention, previously siloed source data becomes directly available for downstream analytics- including purpose-built threat detection tooling.
A purpose-built detection engine should include differentiated capabilities for cutting development time, reducing noise, and improving fidelity. Since the fastest detections to create are the ones you don’t need to build yourself, a threat detection content library is the ultimate timesaver and is out of scope for SOAR vendors.
Also significant are the advanced detection engineering capabilities of purpose-built platforms. For example, multi-stage detection scenarios that identify events of interest in log data and correlate those into threat scenarios. Alerting on threat scenarios, rather than individual atomic detections, is an emerging best practice that results in significantly higher fidelity.
Implementing multi-stage scenario-based detections in a SOAR solution would be cumbersome, if not impossible, at scale. From time-window correlation to grouping indicators by ATT&CK technique, workflow automation does not support detection engineering best practices. With good visibility, SIEM can apply enrichment data and context in an iterative process to avoid false positives and negatives. Detection logic, rather than workflow automation, is the right place to address alert fidelity.
There’s also a data angle to consider. Events of interest, as shown in the second tier below, are fertile ground for threat hunters and data scientists to connect the dots in patterns that don’t emerge from raw events. Advanced use cases require quality detection output stored back in the data platform. When a team relies on downstream automation to sort through the noise, they take on new visibility gaps around activity patterns in the environment. This will be an increasingly important SOC design principle as a wave of AI-powered triage automation solutions hits the shelves.
The relationship between SIEM and SOAR is too often a case of “garbage in, garbage out.” Fixing that requires detection engineering maturity, where high-fidelity alerts set up the automation solution for success. Reliable detection output can be enriched by the SOAR and routed across the organization to reduce the pressure on the SOC and enable self-service remediation. By avoiding SOAR abuse, investments in security automation can go further, and the potential of hyperautomation can be unleashed.